Introduction
- Leveraging ChatGPT for Market Research
- Streamlining Data Analysis with AI
Part 1: Categorising Open-Text Responses with ChatGPT
- Categorising Open-Text Responses
- Rules for Using ChatGPT-3.5
- The Prompts
Part 2: Analysing Open-Text Survey Results with GPT-4 for Premium Account Holders
- Section A: Insights Document Summary some text
- Rationale
- The Prompt
- Example Run
- Section B: Steps to Using GPT-4 for Detailed Analysis of open text
- Segmentation & Cross-Sectional Analysis Prompt
- Correlation Analysis Prompt
- Extraction of Key Insights Prompt
- Summary Statistics of Survey Responses Prompt
- Section C: Graphs and Visualisation Prompts some text
- Graphs and Visualisation prompt
- Visualisation and Insights prompt.
Conclusion
- AI’s role in market research
- Future Implications
FAQ: Using AI for categorisation and analysis of open text response
- How ChatGPT Helps in Categorising Open-Text Survey Responses
- Advanced Insights with GPT-4
- Steps to Prepare Data for AI-Driven Analysis
- Limitations of Using AI for Survey Analysis
- Ensuring Privacy of Survey Data with AI Tools
Introduction
You might be cutting yourself short on ChatGPT’s capabilities for market research. You could have saved yourself hours by leveraging AI to analyse all your raw data for you.
Therefore, with this guide, we aim to help you regain some of the time you would normally spend analysing and spend more time on report writing and communicating the insights you have gathered
This blog consists of two parts. Part A outlines the process for categorising open-text responses with ChatGPT, detailing steps from data preparation to integrating categorised data into broader analyses. It's designed for those seeking to understand respondent feedback at a granular level.
Part B advances to analysing these responses with GPT-4 (for premium account holders), emphasising the AI's ability to handle large datasets and generate insightful visualisations. This section is for researchers ready to dive deeper, offering a pathway to comprehensive insights and strategic decision-making.
Part 1: Open Text Analysis with ChatGPT through copy and pasting
Part 1 serves as a guide for researchers looking to utilise ChatGPT-3.5 for categorising open-text responses collected from surveys or questionnaires. With the integration of AI, it is now possible to efficiently process and categorise qualitative data, providing insightful analysis and a structured overview of respondent feedback. This guide outlines the steps necessary to generate and apply categories to open-text responses using ChatGPT, enabling researchers to incorporate these findings into broader data analysis efforts.
Rules for Using ChatGPT for Categorising Open Text Responses
Rule #1: Generating Categories
- Prepare Your Data: Compile a sample of 50-100 open-text responses to a specific survey question. This number ensures the quality of categorisation.
- Initial Prompt to ChatGPT: Present the sample responses to ChatGPT along with the survey question. Ask for a list of 6-12 categories that are relevant to the question, adhering to the guidelines provided for specificity, sentiment indication, and mutual exclusivity.
- Refine Categories: Review the suggested categories for any necessary refinements or adjustments to better match the themes within the responses.
Rule #2: Categorising Answers
- Prepare the Prompt: Once categories are finalised, prepare a new prompt for ChatGPT that includes the categories and the same set of responses.
- Inclusion of Categories in Prompt: Always include the list of categories within the prompt to ensure ChatGPT does not stray from the established context.
- Coding Responses into Categories: Ask ChatGPT to code each response into the relevant category or categories. This should be done in a table format, with the first column displaying the response and subsequent columns displaying the categories.
- Quality Check: Perform a quality check on the categorised responses to ensure accuracy and completeness. Adjustments may be necessary if responses have been miscategorised.
- Integration into Data Analysis: The final categorised data can then be copied and pasted into an Excel or Google Sheets document for further analysis or integration into larger data sets.
Notes
- Quality Preservation: Limiting responses to 50 at a time helps maintain the quality of categorisation. If more responses need processing, repeat the process in batches.
- Context Preservation: Including the categories in the prompt for the coding phase prevents ChatGPT from generating unintended categories and ensures consistency.
- Continuous Improvement: The categorisation framework may require ongoing adjustments as more data is processed. This iterative process ensures that the categorisation remains relevant and accurate over time.
- UK Spelling: Ensure all categories and prompts use UK English spelling to maintain consistency across the dataset.
Answer Category Creation Prompt
Below is a list of answers to an open-text survey question. Respond with 6-12 categories, depending on how many are needed to code answers into.
Guidelines:
Don't create a category for test data
- Specificity: Refine broad categories, e.g., “Financial Stability” into "Income Stability", "Expense Management", and "Financial Planning".
- Singular Terminology: Use singular terms, e.g., “Girl” instead of “Girls”.
- Sentiment Indication: Indicate sentiment, e.g., “Stable Family” and “Unstable Family” instead of “Family Stability”.
- Open-ended Categorisation: Don't limit the number of categories. Create as many as necessary to cover the themes in the responses.
- Mutual Exclusivity: Ensure categories are mutually exclusive, e.g., “Academic Achievement” and “Career Advancement” instead of a broad “Success” category.
- Multi-categorisation: Code responses into multiple categories if they touch on several themes.
- Consistency: Keep a consistent approach in categorisation, with clear definitions for each category.
- Custom Categories: Create custom categories for unique or uncommon themes.
- Continuous Improvement: Revisit and refine the categorisation framework as needed.
- UK Spelling: Return categories in UK spelling
-- INSERT ANSWERS BELOW –
Example: Category creation prompt response
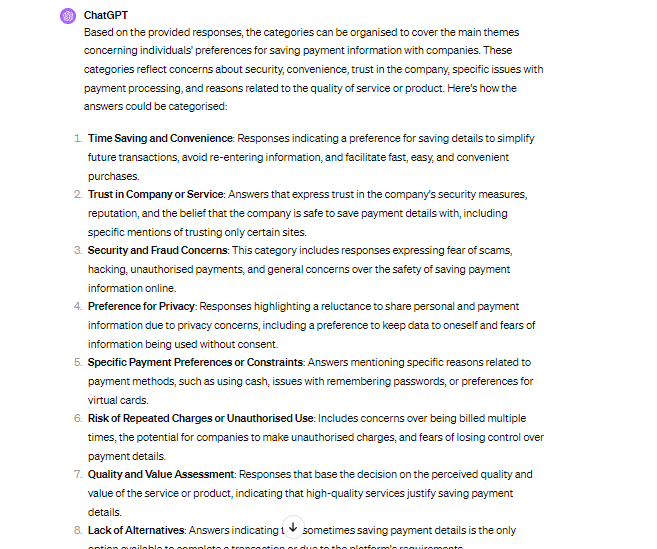
Categorising the answers prompt:
Given the following answers (below), identify the relevant categories they belong to by coding them into the relevant categories (displayed below).
Give back in a table format with the response in the first column and the category in the 2nd column.
If the response fits into more than 1 category, display the category word in the category column for the given row. I.e. the answer category column will only contain the category name in rows where it is categorised and in the heading column.
Answers:
– insert answers below –
Categories:
– insert categories below –
Example: Categorising the answers prompt response
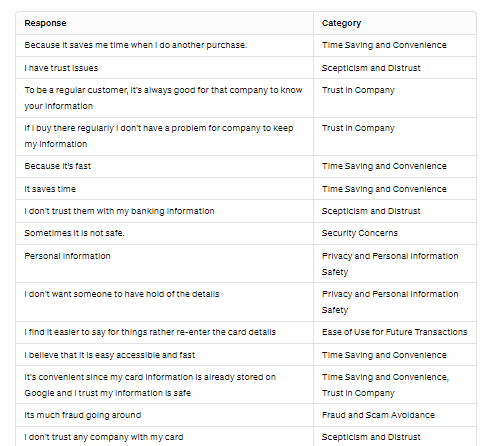
Part 2: Data Analysis Prompts for creating a 3-page insights doc summary with ChatGPT
Part 2 is segmented into different sections to provide a clear guide for premium account holders utilising ChatGPT for analysing open-text survey results. Sections A and B focus on summarising, segmenting, and conducting cross-sectional analysis, while Section C emphasises the generation of graphs and visualisations from the open-text responses. By harnessing AI capabilities, users can upload datasets directly and efficiently extract meaningful insights and visualizations. This guide offers detailed instructions on navigating this advanced feature, ensuring researchers can interpret qualitative data effectively and integrate insights into their analysis strategy.
Notes:
- Quality Preservation: Utilising a Data Analyst GPT ensures analysis quality. Clear research objectives are essential for determining analysis quality.
- Context Preservation: Review GPT-4 results for relevance to prevent unintended analysis. Tailor comparative analysis to specific segmentation needs to avoid token limit constraints.
- Continuous Improvement: Regularly adjust the categorisation framework to maintain relevance and accuracy as more data is processed.
Section A: Data Analysis Prompts for creating a 3-page insights doc summary with ChatGPT
Rationale:
This prompt is for you to gain a summary of the answers received, the insights you can draw from them, segmenting and what strategy you might employ with the insights given. This is going to give you a leg forward when it comes to reporting your findings to your clients.
Notes:
- Be mindful of token limitations. Break prompts into smaller sections to prevent hallucinations.
- The end of each prompt is marked by
Prompt intro:
You are a market research and consumer insights expert with exceptional data analysis skills for gleaning insights that others aren't able to see.
I need to analyze this survey response dataset from the dataset uploaded about {what your research was about/ your research objective}. I want to write a snapshot report for this project with a summary of the key findings, insights, and themes. Can you help me?
Rules you should abide by:
- UK English
- Give insights in bullets
- Use actual statistics whenever referencing anything or making a point. Always use data when making a point - both % and response count!
- bold key stats and points
First, analyse these questions/columns in the dataset before I give you the actual dataset and figure out your analysis approach in terms of which questions need to be analysed for the different future prompts (see below).
You will be analysing and giving insights over 3 prompts so make sure to identify the question columns and analysis strategies for each of the 3 prompt categories below
Prompt 1: Summary and overall insights
- Executive summary of key insights and themes in a short paragraph
- Key insights that came through, considering the objective of the survey, were broken into different relevant sections for the survey. In bullet form and backup points with stats and percentages
- Go beyond the obvious findings and analyse my survey data to reveal the top 3 most surprising or unexpected insights. What hidden gems are lurking in the responses?
Example: Summary and overall insights prompt response
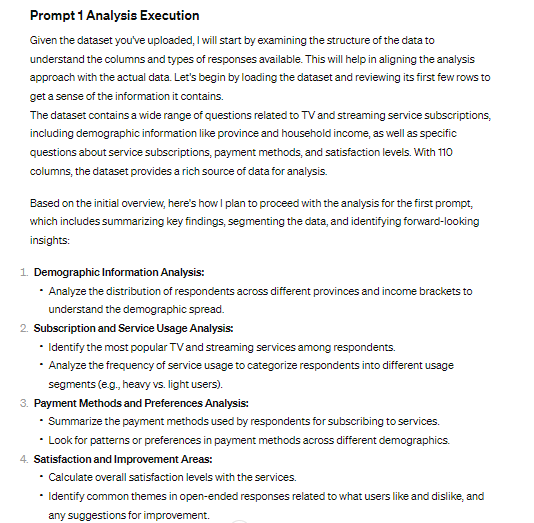
Prompt 2: Segmenting
- Analyse my survey data to identify any significant differences in responses across demographic groups (age, income, location, etc.). Are there any key trends or patterns that emerge? and summarise the key differences in opinions or preferences among these groups?"
- Comparative Analysis: "Compare the responses from different segments (e.g., high vs. low frequency or consumption respondents) and highlight any significant differences."

Prompt 3: Forward-looking
- Based on the survey responses, suggest potential customer segments with distinct characteristics and needs. How can I use this information to tailor my marketing and product offerings?
- Based on the analysis of my survey data, provide actionable recommendations for the client in terms of marketing, product, and growth strategies. What are the specific steps I can take to implement these insights?
- Analyse my survey data to identify the biggest unaddressed needs and desires expressed by respondents. Is there a potential market opportunity I can capitalise on?
Now complete the analysis for prompt 1 with your approach from above and my guidelines below:
Example: Forward-looking prompt response
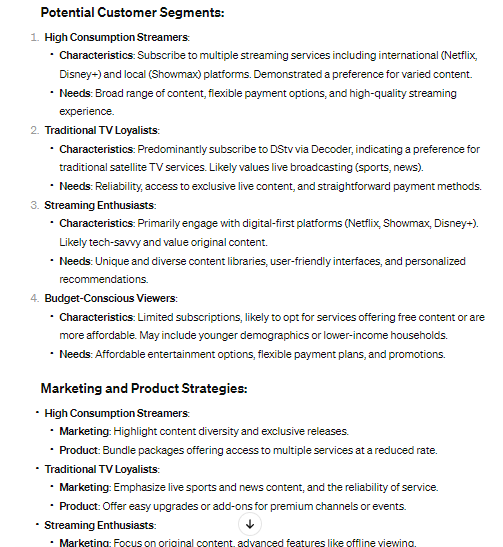
Section B: Helpful prompts for analysing different aspects of the data
Segmentation & Cross-Sectional Analysis Prompt
Rationale: These prompts segment data into groups and compare responses between them.
Prompt 1:
- “Can you segment the data into meaningful groups based on respondent demographics or the below question? Then, analyse the differences in responses between these groups." {insert Question}
- Please analyse the groups across other answers in a proportionate way and not by just counting how many answered x within a group. This enables us to do a fair comparison if there is an uneven split between the count of respondents in the group.
Example: Segmentation & Cross-Sectional Analysis Prompt 1 response
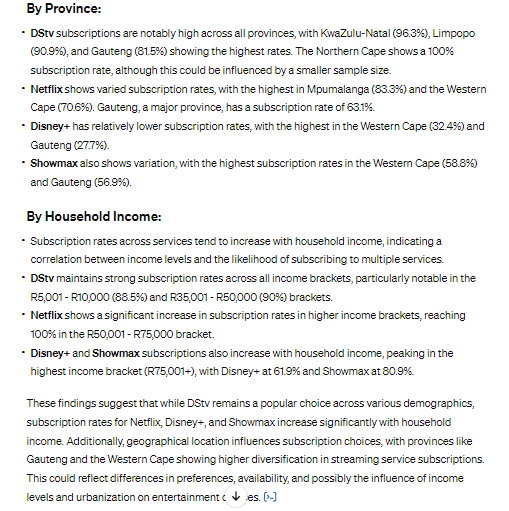
Prompt 2:
"Please divide the survey data into relevant segments, such as by age or gender. Then, compare the responses across these segments."
Example: Segmentation & Cross-Sectional Analysis Prompt 2 response
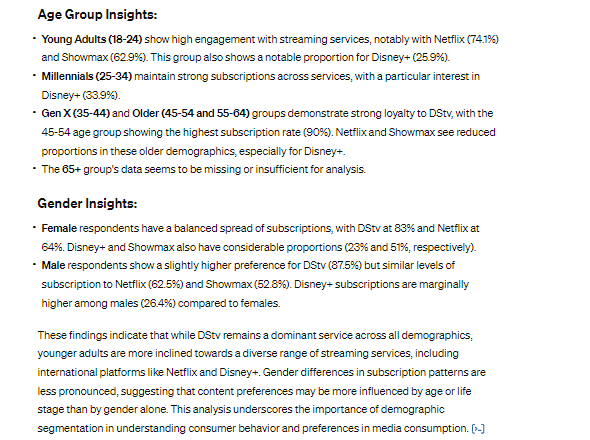
Prompt 3:
I'm looking for a way to segment [data set] into different groups based on [criteria] and analyse the differences between them.
Example: Segmentation & Cross-Sectional Analysis Prompt 3 response
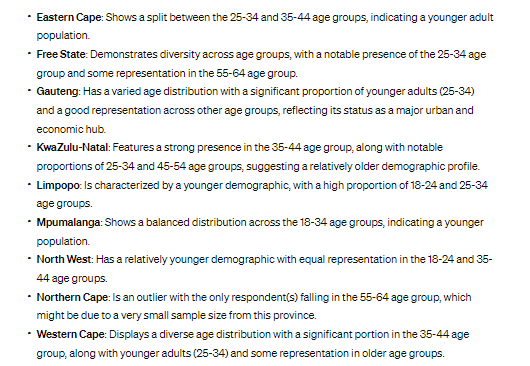
Correlation Analysis Prompt
Rationale: This prompt identifies correlations between different questions or segments of the survey data.
Prompt 1:
"Could you analyse the survey data for correlations? Identify any significant relationships between different answers and segments."
Example: Correlation Analysis Prompt response
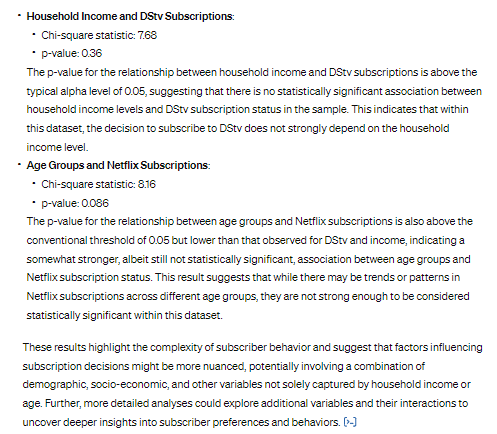
Prompt 2:
"Please examine the survey data for correlations. I'm interested in any strong relationships between different responses or segments."
Extraction of Key Insights Prompt
Rationale: This prompt aims to derive key insights from the data, focusing on correlations and trends.
Prompt 1:
"Can you derive key insights from the survey data? I'm particularly interested in correlations and trends in the data."
Example: Extraction of Key Insights Prompt response
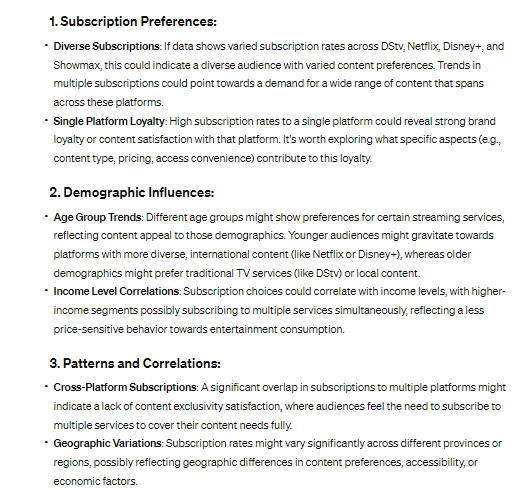
Prompt 2:
"Please provide me with the most significant insights from the survey data, paying special attention to trends and correlations."
Example: Significant Insights Prompt response
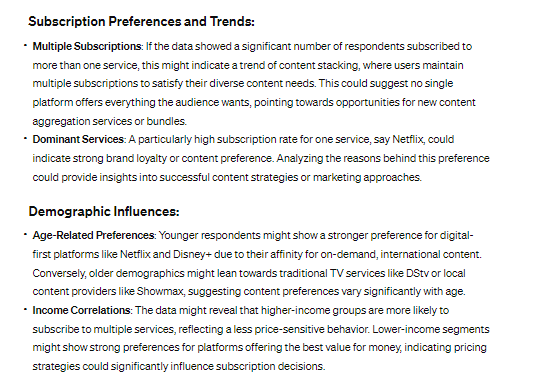
Summary Statistics of Survey Responses Prompt
Rationale: These prompts generate a summary table showing the frequency and proportion of each answer for all questions.
Prompt 1:
"Can you generate a summary table of the survey responses in ’'{insert CSV name}'? For each question, include the count and percentage of each answer option. The question should occupy 3 columns: the question in as the heading with the options below, a column called Count which counts the occurrences, and a column called Proportion which calculates the %.
Each set of question results should be next to each other so there should be 3x the amount of columns that you calculate.
Give the results back to me in a new CSV called '{insert CSV name}'
Prompt 2:
"Please create a summary table for our survey data. This table should show the number and proportion of each response for every question."
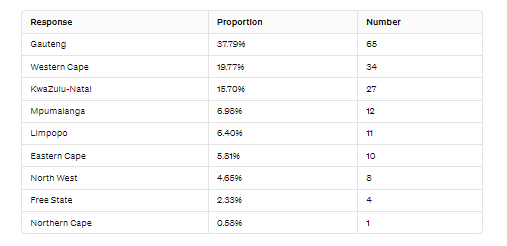
Section C: Graphs & Visualisations Prompts
Graph and Visualisation prompt:
Rationale: This section creates visual representations of the most interesting data points, using specific brand colours.
Prompt 1:
- "Could you create graphs to visualise the most interesting data points from the survey? Please use my brand colours for these visualisations."
- "Please generate visualisations of the most significant data points from the survey. Make sure to incorporate my brand colours in these graphics."
Example: Bar graph of interesting data points

Visualisation and Insights Prompt:
Rationale: This section transforms data' into visualisations, highlighting key insights through graphs and bullet points for clear, actionable understanding.
Prompt:
Analyse '{insert CSV}' and show me 2-3 interesting visualisations with precise insight in 3-4 bullet points
Example: Visualisation and Insights Prompt response
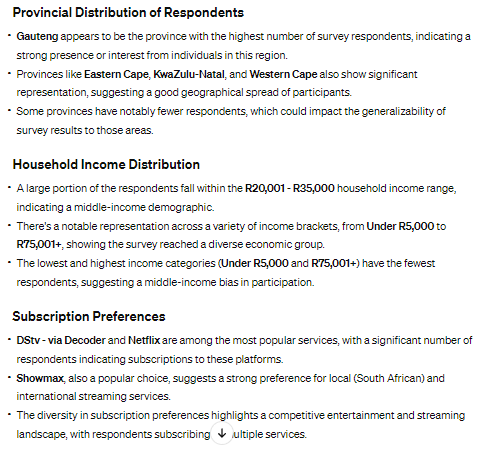
.....
Conclusion
As we wrap up our exploration of survey data through GPT-4's analytical prowess and visual storytelling, it's clear that the integration of AI in market research not only simplifies the data analysis process but also uncovers hidden gems within the dataset. By following the structured approach laid out in this article, researchers and analysts can transform raw data into strategic insights, paving the way for informed decision-making and innovative strategies. The future of data analysis in market research is here, and AI powers it.
FAQ: Using AI for Categorisation and Analysis of Open-Text Responses
How does ChatGPT help in categorising open-text survey responses?
ChatGPT assists by analysing the content of survey responses to suggest or directly classify them into relevant categories. This process simplifies the analysis by organising vast amounts of data into manageable, themed groups.
Can GPT-4 analyse survey data for advanced insights beyond categorisation?
Yes, GPT-4 offers enhanced capabilities for deep analysis, including trend identification, sentiment analysis, and the generation of visualisations, providing comprehensive insights from complex data sets.
What are the steps to prepare data for AI-driven analysis?
Start by cleaning your dataset to remove any irrelevant or duplicate responses. Clearly define your survey questions and objectives. For GPT-4, ensure the data is structured appropriately for the analysis you intend to conduct.
Are there limitations to using AI for survey analysis?
While AI tools like ChatGPT and GPT-4 are powerful, they might not fully capture the nuances of all responses without detailed instructions. The accuracy of the analysis also depends on the quality of the input data and the clarity of the analysis objectives.
How can I ensure the privacy of survey data when using AI tools?
Use anonymised responses whenever possible, and adhere to your AI service provider's data handling and privacy guidelines. Make sure sensitive information is either removed or securely anonymised before analysis.